
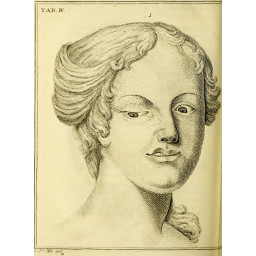
There’s also a whole subreddit from hell about this subgenre of fiction: https://www.reddit.com/r/rational/
/r/rational isn’t just for AI fiction, it also claims includes anything with decent verisimilitude, so stuff like The Hatchet and The Martian show up in its recommendation lists also! letting it claim credit for better fiction than the AI stuff
It’s not all the exact same!
Friendship is Optimal adds in pony sex